Latest Posts
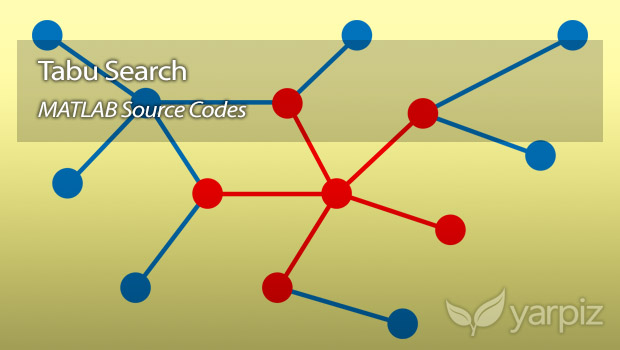
Tabu Search (TS) in MATLAB
Tabu Search (TS) is a local search-based metaheuristic, which is proposed by Fred W. Glover, in 1986. Tabu Search is completely based on the definition of neighborhood and actions converting a solution to its neighboring solutions. This algorithms starts with a single solution, and searches for better solutions,
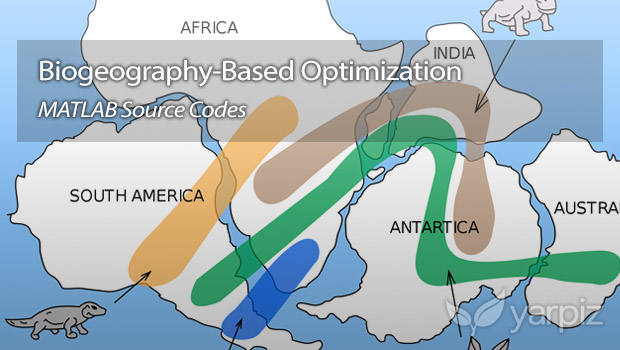
Biogeography-Based Optimization (BBO) in MATLAB
Biogeography-Based Optimization (BBO) is an evolutionary algorithm and metaheuristic, which is inspired by the biogeographic concepts: speciation (the evolution of new species), the migration of species between islands, and the extinction of species. The algorithm is originally proposed by Dan Simon, in 2008, in this paper. This algorithm
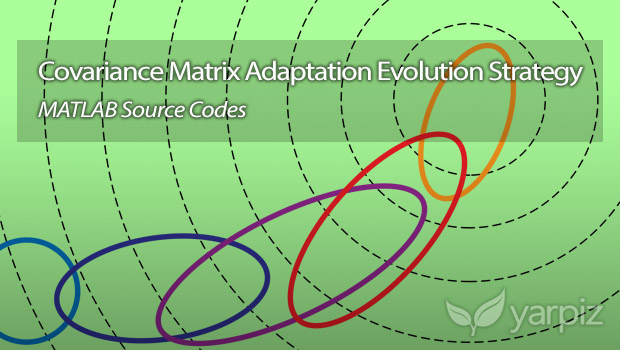
CMA-ES in MATLAB
Evolution Strategy (ES) is the first and oldest evolutionary algorithm, and it is based on the adaptation and evolution. Specially, the main concept used to describe how Evolution Strategy works, is the Evolution of Evolution. In fact, evolution strategy is a family of related algorithms, and because of
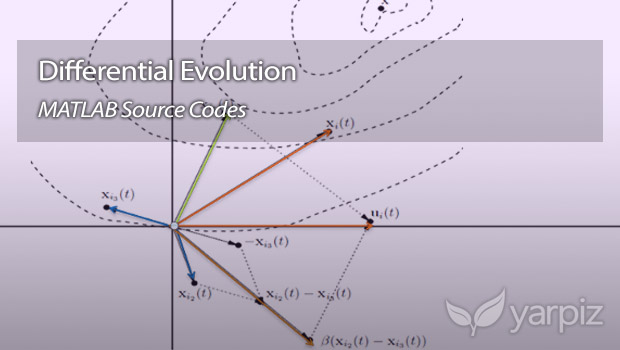
Differential Evolution (DE) in MATLAB
Differential Evolution (DE) is an evolutionary algorithm, which uses the difference of solution vectors to create new candidate solutions. The key points, in the usage of population differences in proposition of new solutions, are: The distribution of population and its orientation is hidden in the differences of population
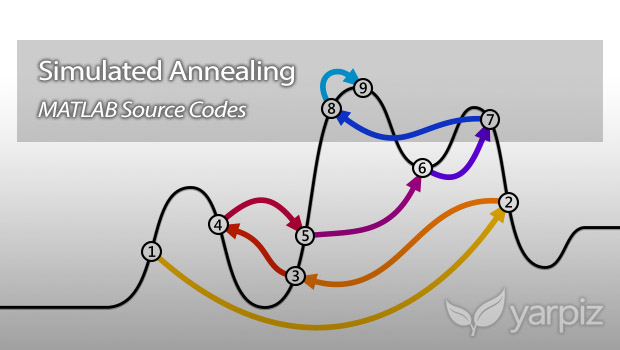
Simulated Annealing in MATLAB
Simulated Annealing (SA) is a metaheuristic, inspired by annealing process. SA starts with an initial solution at higher temperature, where the changes are accepted with higher probability. So the exploration capability of the algorithm is high and the search space can be explored widely. As the algorithm continues
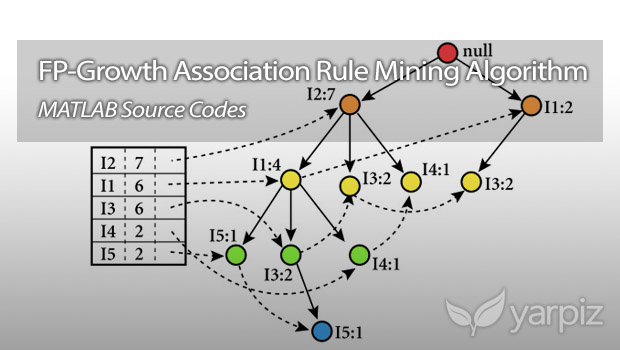
FP-Growth Association Rule Mining in MATLAB
Like Apriori algorithm, FP-Growth is an association rule mining approach. The term FP in the name of this approach, is abbreviation of Frequent Pattern. FP-Growth uses a frequent pattern mining technique to build a tree of frequent patterns (FP-Tree), which can be used to extract association rules. Compared
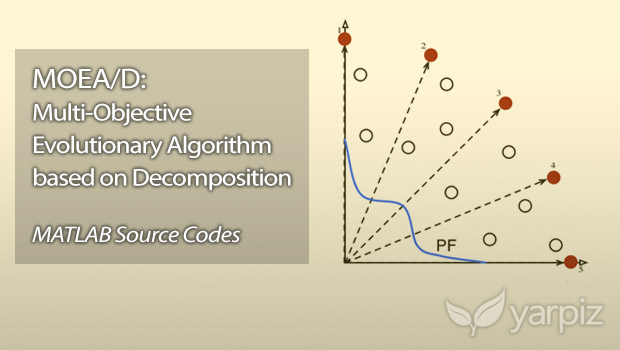
MOEA/D in MATLAB
One of the classic approaches to deal with multi-objective optimization problems, is decomposition, which means that a multi-objective is decomposed to several (theoretically infinite) single-objective optimization problems. Decomposed objective functions, can be defined using several methods, like weighted sum of objectives and distance (or norm) of difference vector
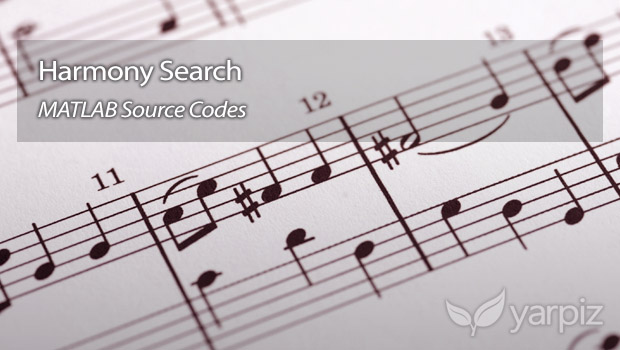
Harmony Search in MATLAB
Harmony Search (HS) is a global optimization algorithm which inspired by harmony improvisation process of musicians, proposed by Zong Woo Geem in 2001. Every solution in this algorithm is called a Harmony and there is an archive of promising solutions, called Harmony Memory (HM). For more information on
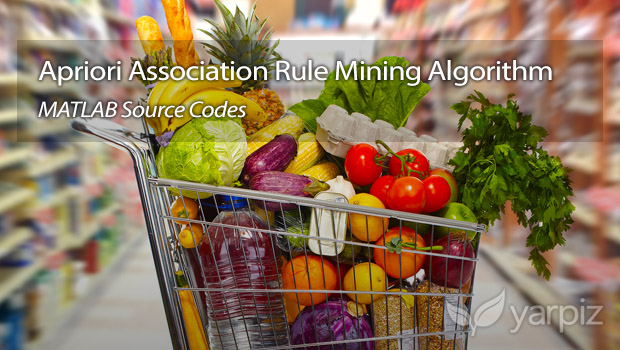
Apriori Association Rule Mining in MATLAB
Association Rule Mining is a common task in the field of Data Mining, involving the recognition of frequent patterns, usually in transactional databases. For example, discovering a rule like {bread, butter} → {milk} in a sales dataset is a result of association rule mining, and indicates that if a
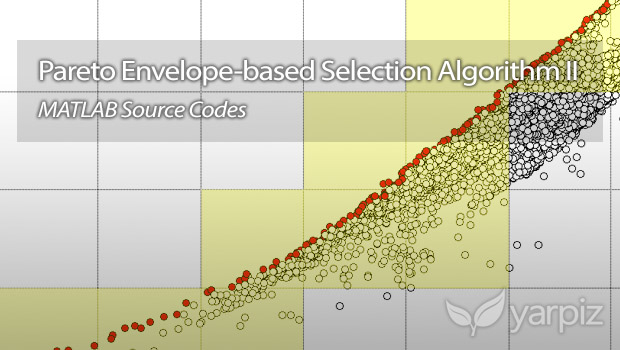
PESA-II in MATLAB
Pareto Envelope-based Selection Algorithm II (PESA-II) is a multi-objective evolutionary optimization algorithm, which uses the mechanism of genetic algorithm together with selection based on Pareto envelope. PESA-II uses an external archive to store the approximate Pareto solutions. Parents and mutants are selected from this external archive, based on
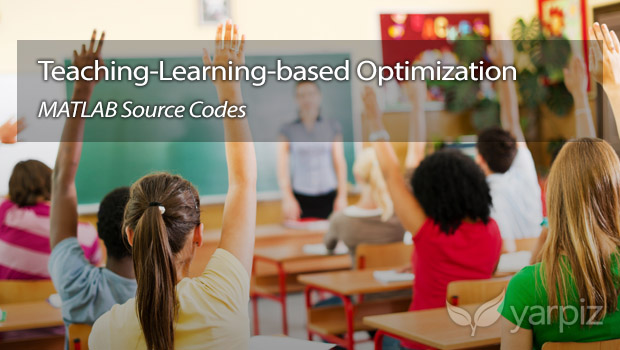
Teaching-Learning-based Optimization in MATLAB
Teaching-Learning-based Optimization (TLBO) is a metaheuristic, inspired by process of Teaching and Learning, via a simplified mathematical model of knowledge improvements gained by students in a class. This algorithm is proposed by Rao, Savsani and Vakharia in 2011, in this paper. In this post, we are going to
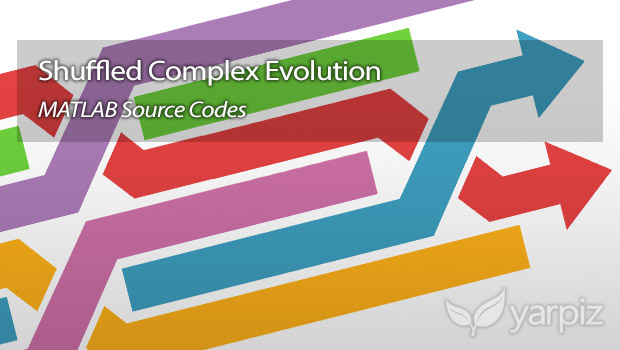
Shuffled Complex Evolution in MATLAB
Shuffled Complex Evolution (SCE-UA) is a metaheuristic for global optimization, proposed by Duan, Gupta and Sorooshian, in 1992. Because SCE is the abbreviated name of other methods in the science, the UA is added to the abbreviated name of this algorithm, because the creators of this algorithm are
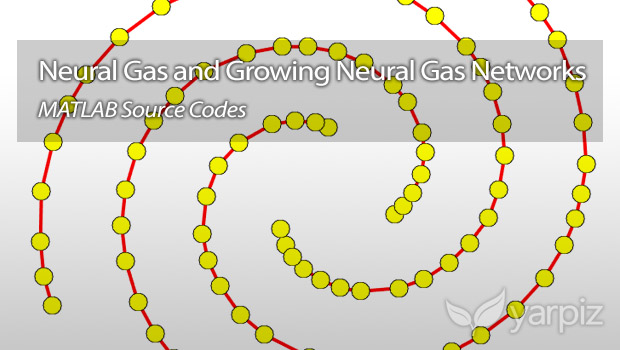
Neural Gas and GNG Networks in MATLAB
Neural Gas network is a competitive Artificial Neural Network (ANN), very similar to Self-Organizing Map (SOM), which is proposed by Martinetz and Schulten, 1991. Neural Gas network can be used to solve unsupervised learning tasks, like clustering, dimensionality reduction, and topology learning. It has many applications in the
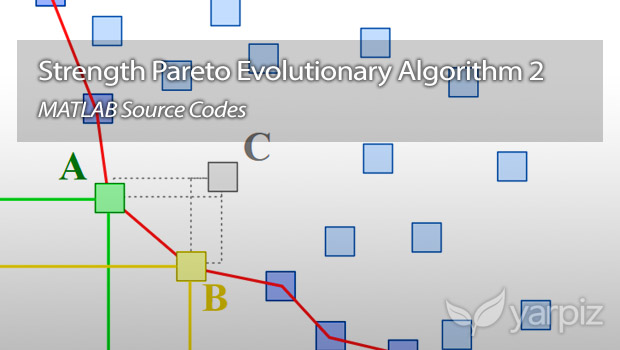
Strength Pareto Evolutionary Algorithm 2 (SPEA2) in MATLAB
Strength Pareto Evolutionary Algorithm 2 (SPEA2) is an extended version of SPEA multi-objective evolutionary optimization algorithm. This algorithm utilized a mechanism like k-Nearest Neighbor (kNN) and a specialized ranking system to sort the members of the population, and select the next generation of population, from combination of current
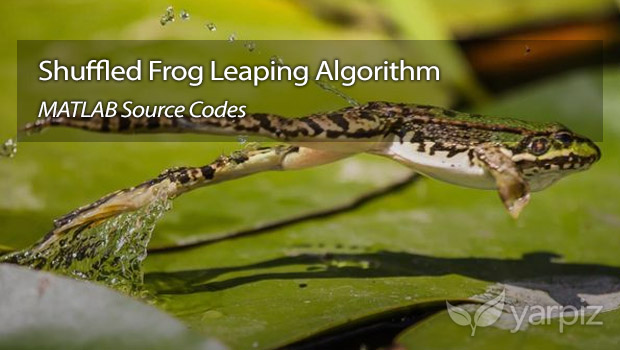
Shuffled Frog Leaping Algorithm in MATLAB
Shuffled Frog Leaping Algorithm (SFLA) is a metaheuristic, or more accurately it is a Memetic Algorithm, which is inspired by frog leaping. SFLA is based on the model used by Shuffled Complex Evolution (SCE-UA), and incorporated the memetic evolution into it. It is applicable to any kind of
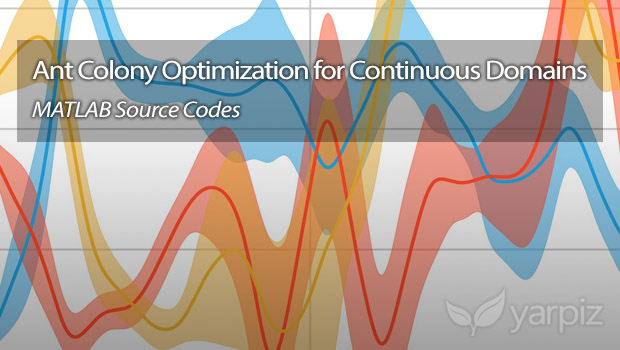
ACO for Continuous Domains in MATLAB
Originally, the Ant Algorithms are used to solve discrete and combinatorial optimization problems. Various extensions of Ant Colony Optimization (ACO) are proposed to deal with optimization problems, defined in continuous domains. One of the most useful algorithms of this type, is ACOR, the Ant Colony Optimization for Continuous
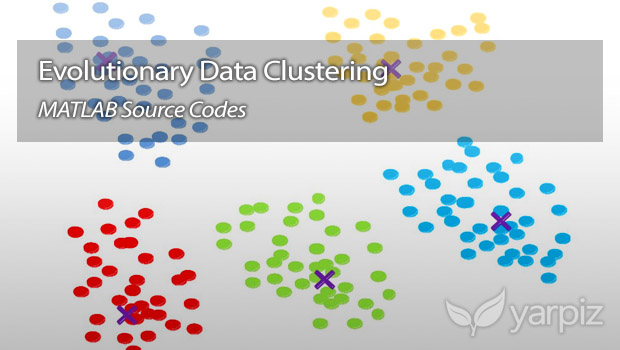
Evolutionary Data Clustering in MATLAB
Clustering is an unsupervised machine learning task and many real world problems can be stated as and converted to this kind of problems. Clustering is grouping a set of data objects is such a way that similarity of members of a group (or cluster) is maximized and on
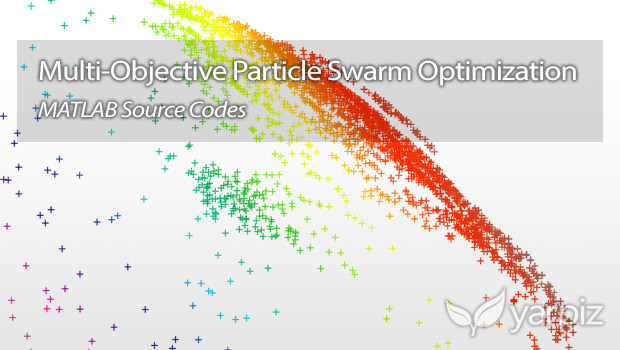
Multi-Objective PSO in MATLAB
Multi-Objective Particle Swarm Optimization (MOPSO) is proposed by Coello Coello et al., in 2004. It is a multi-objective version of PSO which incorporates the Pareto Envelope and grid making technique, similar to Pareto Envelope-based Selection Algorithm to handle the multi-objective optimization problems. Just like PSO, particle in MOPSO
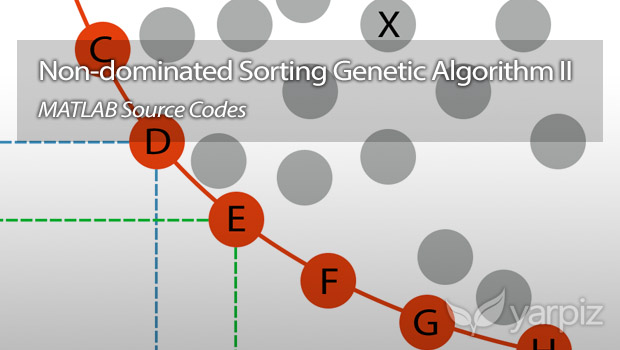
NSGA-II in MATLAB
Non-dominated Sorting Genetic Algorithm II (NSGA-II) is a multi-objective genetic algorithm, proposed by Deb et al., in 2002. It is an extension and improvement of NSGA, which is proposed earlier by Srinivas and Deb, in 1995. In the structure of NSGA-II, in addition to genetic operators, crossover and
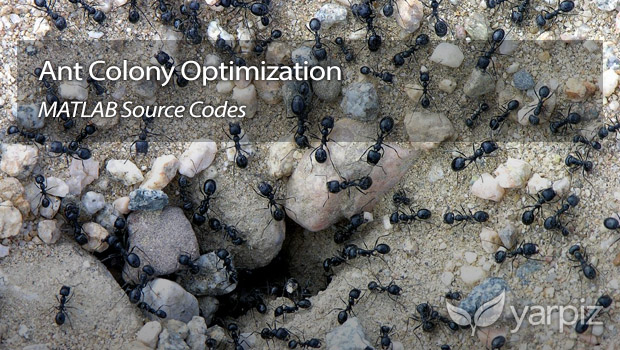
Ant Colony Optimization in MATLAB
Ant Colony Optimization (ACO) are a set of probabilistic metaheuristics and an intelligent optimization algorithms, inspired by social behavior of ants. ACO algorithms are also categorized as Swarm Intelligence methods, because of implementation of this paradigm, via simulation of ants behavior in the structure of these algorithms. First
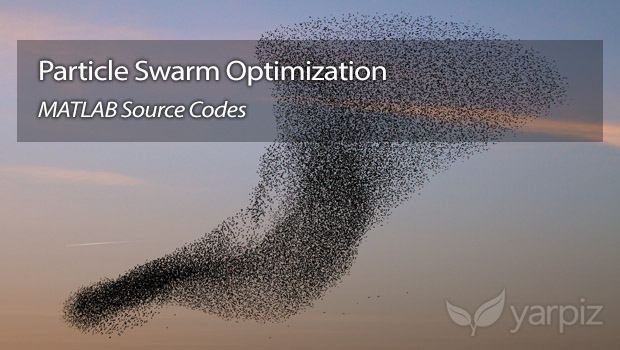
Particle Swarm Optimization in MATLAB
[box type=”info” ]A video tutorial on PSO implementation in MATLAB is freely available for download, in this link.[/box] Particle Swarm Optimization (PSO) is an intelligent optimization algorithm based on the Swarm Intelligence. It is based on a simple mathematical model, developed by Kennedy and Eberhart in 1995, to
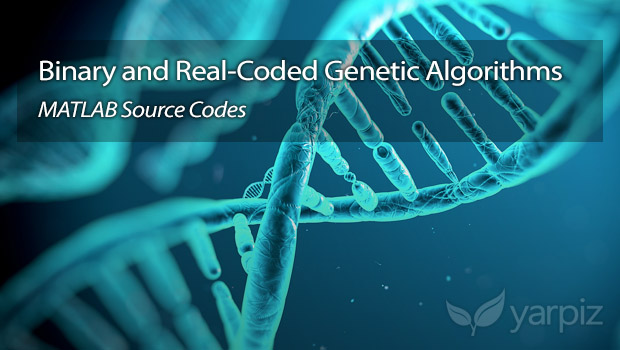
Binary and Real-Coded Genetic Algorithms in MATLAB
Genetic Algorithms (GAs) are most famous Evolutionary Algorithms (EAs) which are inspired from natural evolution and selection. Their main application is in the field of optimization. Hence they are applicable to any kind of problem, which can be converted or stated as an optimization task. To read more